In part 1 of our Series on Data Science for Utilities, we explored the basics, and in part 2, the benefits. Part 3 will review the importance of working with cleansed data for utilities to adapt to the new demands accompanying the energy transition the industry is facing.
Data cleansing identifies and corrects or removes dataset errors, inconsistencies, and inaccuracies.
So let’s break it down – why is data cleansing important?
It enables enhanced data analysis:
Data cleansing can improve the accuracy and reliability of data analysis by removing or correcting errors that can lead to incorrect conclusions. Data scientists need to have confidence in the quality of their data before they can perform meaningful analyses.
It saves time & resources:
Data cleansing can save time and resources by automating the identification and correction of errors rather than manually checking and correcting each record in the dataset. This allows data scientists to focus on the analysis rather than spending time on data cleansing.
It reduces risks & costs:
Data cleansing can reduce the risks and costs associated with inaccurate or inconsistent data. For example, using inaccurate data for decision-making can lead to poor decisions, increased costs, and damage to a utility’s reputation.
Ensures compliance:
Data accuracy and reliability are essential for protecting the privacy of individuals and avoiding legal liabilities.
Despite data cleansing being essential in making the insights gleaned from data reliable, it presents a massive hurdle for utilities. This is due to several reasons, including:
- Large data volumes: Utility data can be massive, with millions of data points generated daily. Cleaning such vast volumes of data can be time-consuming and resource-intensive.
- Data complexity: Utility data can have various types and formats. It may require specialized knowledge and tools to identify and address anomalies.
- Incomplete or missing data: Utility data can contain incomplete or missing data, which can be challenging to address. It may require data imputation techniques, which can be complex and prone to errors.
- Data integration challenges: Utility data may come from multiple sources, such as smart meters, sensors, and other devices. Integrating this data can be challenging, requiring specialized tools and techniques.
- Data security concerns: Utility data may contain sensitive customer information, making it essential to maintain data privacy and security while cleaning the data.
Overcoming these challenges and obtaining accurate and valuable insights from utility data is possible with the right tools, techniques, and expertise. Such a tool exists within the Energy Transition Platform – The AI Data Engine. The Awesense AI Data Engine uses sophisticated algorithms to cleanse, structure, and synchronize time-series data (AMI/AMR, SCADA, Sensors, etc.) with GIS data according to the Awesense Open Energy Data Model (EDM), resulting in a digital twin of a utility’s grid. The AI Data Engine’s key features are its AI-driven validation, estimation, and error correction capabilities.
Types of corrections the AI Data Engine can perform:
- Geo-coordinates validation and transformation
- Connectivity validation, estimation and correction
- Meter association validation and correction
- Switch state validation and correction
- Time series data validation and synchronization
- Missing time series data estimation
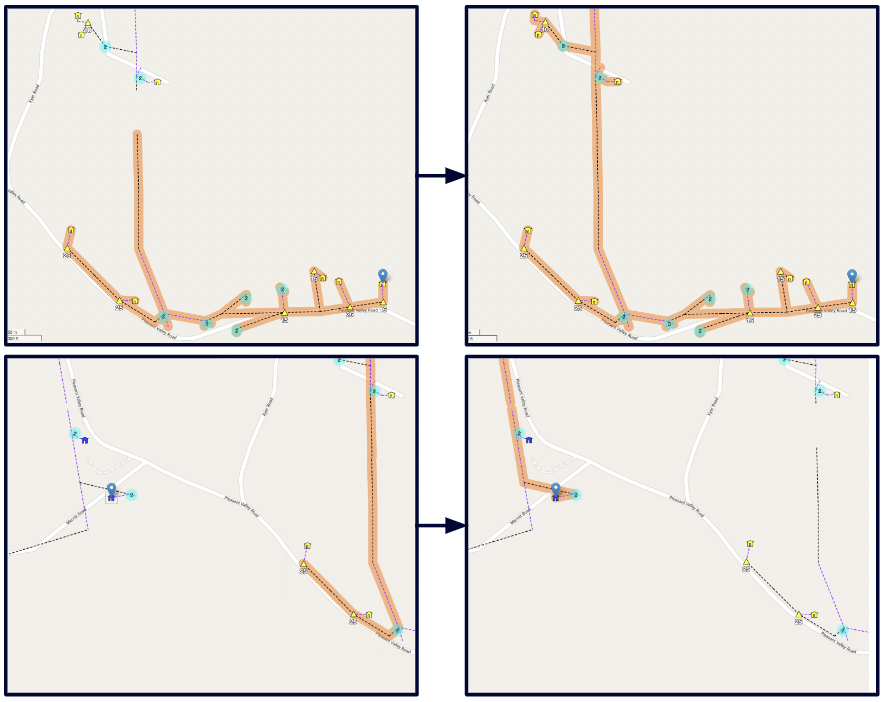
In summary, data cleansing is a hurdle for many utilities, impeding their ability to use data science to its full analytical potential. However, we are here to tell you that there are solutions!
We are passionate about the energy transition and believe the path forward is digitalization. We have developed tools to help organizations digitalize and unlock the power of data. Contact us directly for more information on our AI Data Engine and Data Science Services.